Nonparametric methods
Nonparametric Tests Wilcoxon Mann-Whitney Test Wilcoxon Signed Ranks Test Sign Test Runs Test Kolmogorov-Smirnov Test Kruskal-Wallis Test |

Nonparametric tests are often used in place of their parametric counterparts when certain assumptions about the underlying population are questionable. For example, when comparing two independent samples, the Wilcoxon Mann-Whitney test does not assume that the difference between the samples is normally distributed whereas its parametric counterpart, the two sample t-testdoes. Nonparametric tests may be, and often are, more powerful in detecting population differences when certain assumptions are not satisfied.
All tests involving ranked data, i.e. data that can be put in order, are nonparametric.

The Wilcoxon Mann-Whitney Test is one of the most powerful of the nonparametric tests for comparing two populations. It is used to test the null hypothesisthat two populations have identical distribution functions against the alternative hypothesisthat the two distribution functions differ only with respect to location (median), if at all.
The Wilcoxon Mann-Whitney test does not require the assumption that the differences between the two samples arenormally distributed.
In many applications, the Wilcoxon Mann-Whitney Test is used in place of the two sample t-test when the normality assumption is questionable.
This test can also be applied when the observations in a sample of data are ranks, that is,ordinal data rather than direct measurements.

The Wilcoxon Signed Ranks test is designed to test a hypothesis about the location (median) of a population distribution. It often involves the use of matched pairs, for example, before and after data, in which case it tests for a median difference of zero.
The Wilcoxon Signed Ranks test does not require the assumption that the population is normally distributed.
In many applications, this test is used in place of the one sample t-test when the normality assumption is questionable. It is a more powerful alternative to the sign test, but does assume that the population probability distribution is symmetric.
This test can also be applied when the observations in a sample of data are ranks, that is, ordinal data rather than direct measurements.

The sign test is designed to test a hypothesis about the location of a population distribution. It is most often used to test the hypothesis about a population median, and often involves the use of matched pairs, for example, before and after data, in which case it tests for a median difference of zero.
The Sign test does not require the assumption that the population isnormally distributed.
In many applications, this test is used in place of the one sample t-test when the normality assumption is questionable. It is a less powerful alternative to theWilcoxon signed ranks test, but does not assume that the population probability distribution is symmetric.
This test can also be applied when the observations in a sample of data are ranks, that is, ordinal data rather than direct measurements.

In studies where measurements are made according to some well defined ordering, either in time or space, a frequent question is whether or not the average value of the measurement is different at different points in the sequence. The runs test provides a means of testing this.
Example
Suppose that, as part of a screening programme for heart disease, men aged 45-65 years have their blood cholesterol level measured on entry to the study. After many months it is noticed that cholesterol levels in this population appear somewhat higher in the Winter than in the Summer. This could be tested formally using a Runs test on the recorded data, first arranging the measurements in the date order in which they were collected.

For a single sample of data, the Kolmogorov-Smirnov test is used to test whether or not the sample of data is consistent with a specified distribution function. When there are two samples of data, it is used to test whether or not these two samples may reasonably be assumed to come from the same distribution.
The Kolmogorov-Smirnov test does not require the assumption that the population is normally distributed.
Compare Chi-Squared Goodness of Fit Test.

The Kruskal-Wallis test is a nonparametric test used to compare three or more samples. It is used to test the null hypothesis that all populations have identical distribution functions against the alternative hypothesis that at least two of the samples differ only with respect to location (median), if at all.
It is the analogue to the F-test used in analysis of variance. While analysis of variance tests depend on the assumption that all populations under comparison are normally distributed, the Kruskal-Wallis test places no such restriction on the comparison.
It is a logical extension of theWilcoxon-Mann-Whitney Test.
Top of page | Main Contents page
cek kursus kami




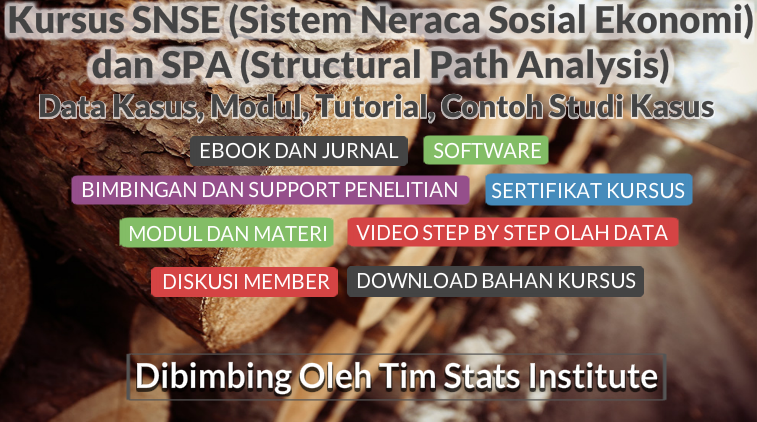
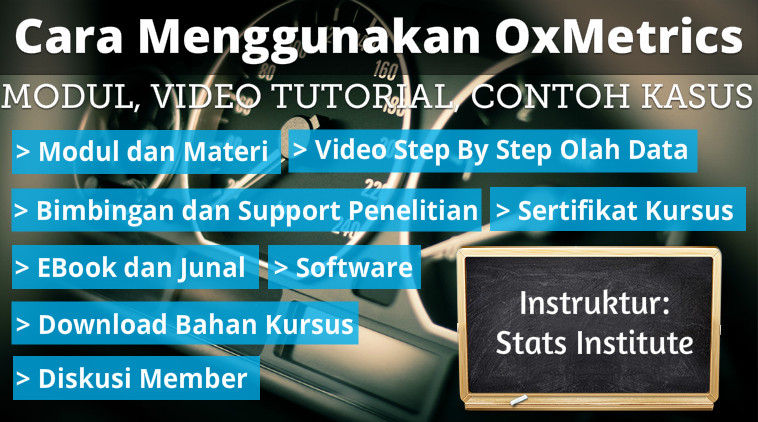
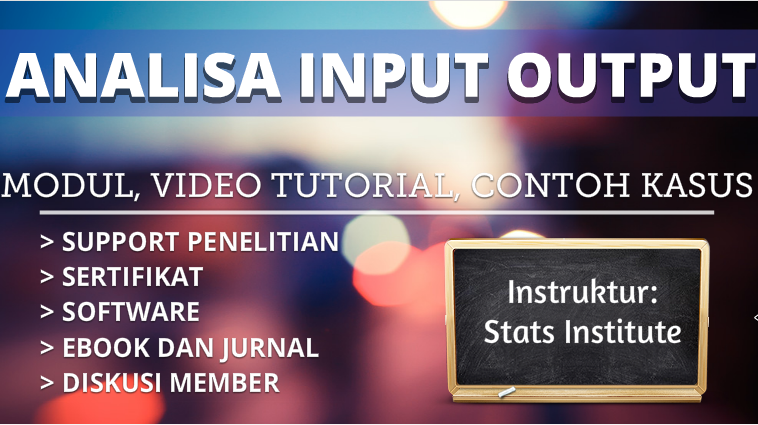

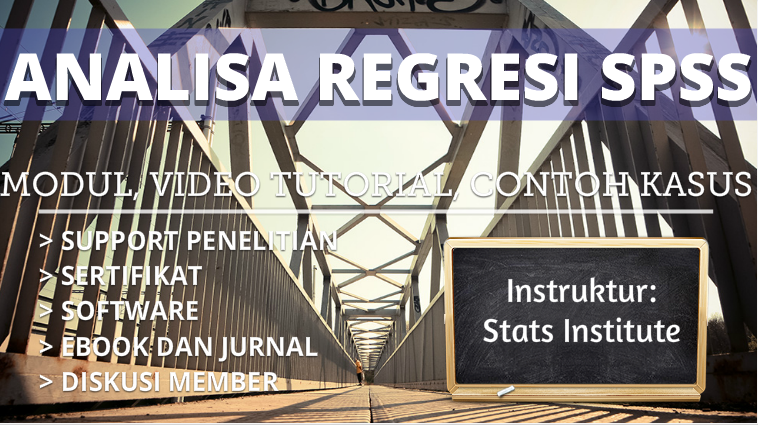

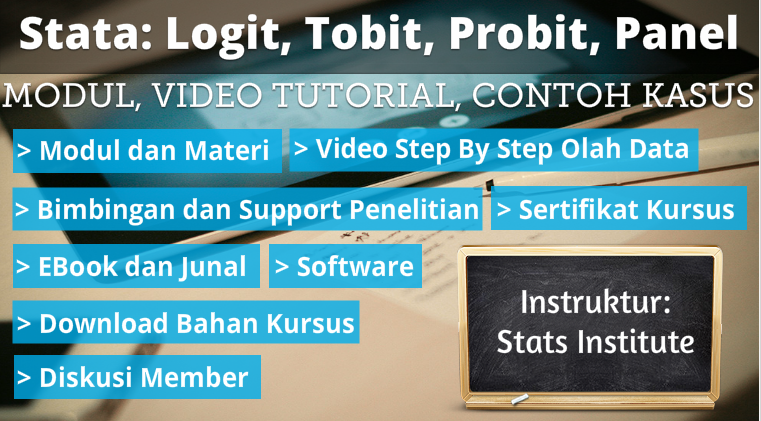

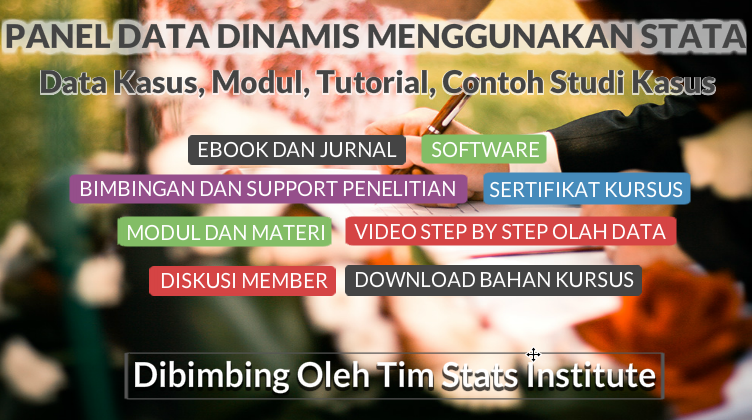
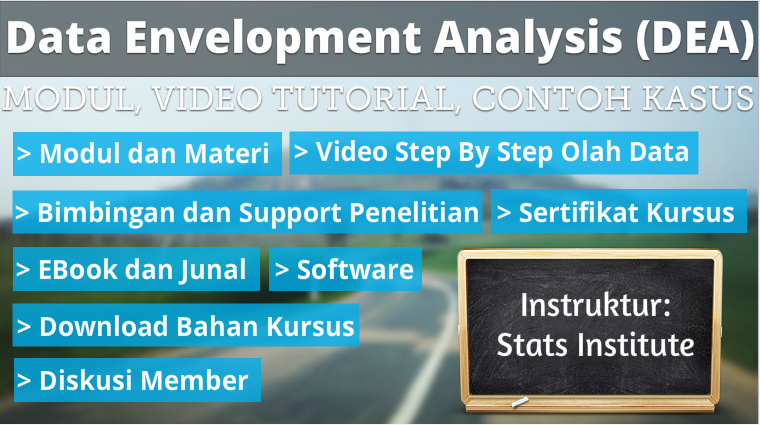

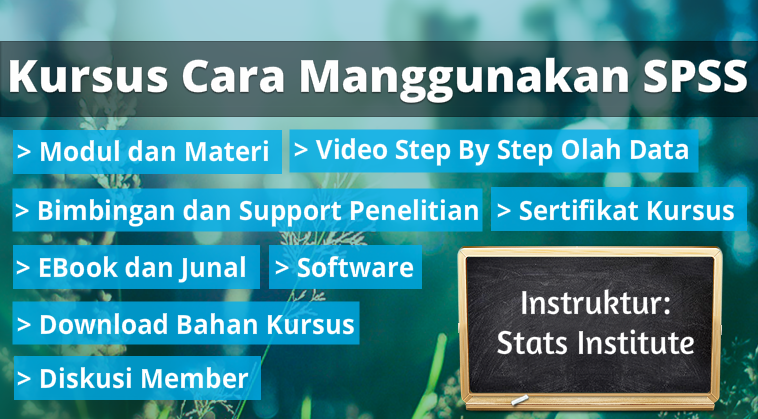

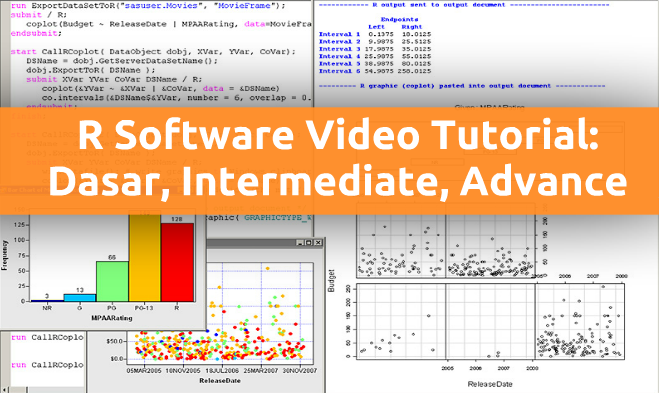
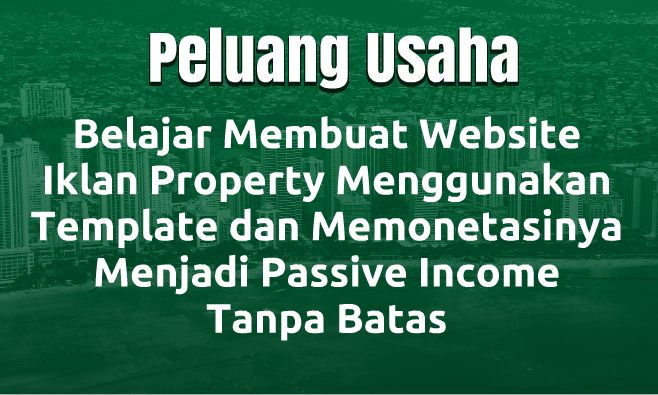
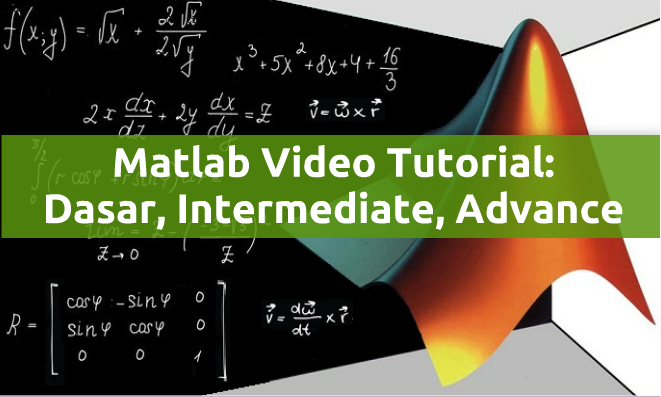


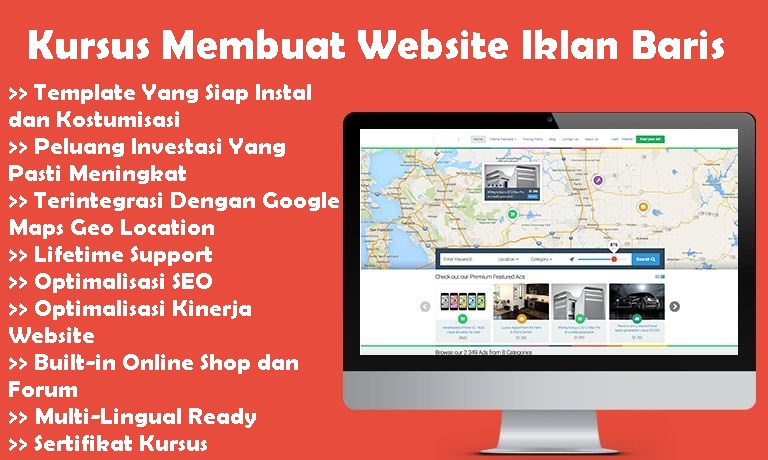
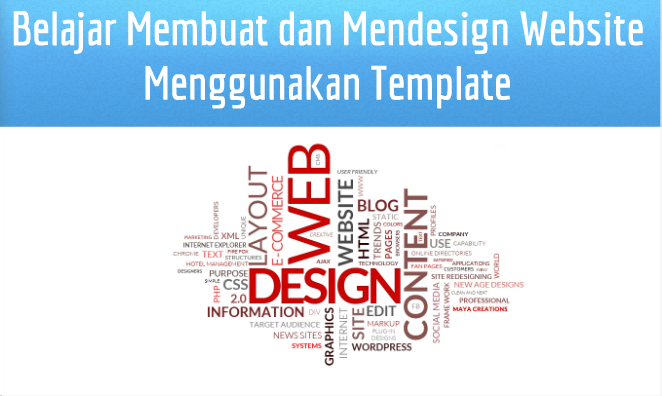
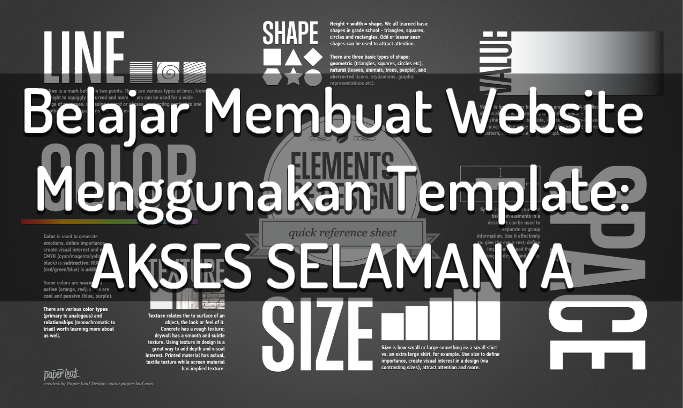


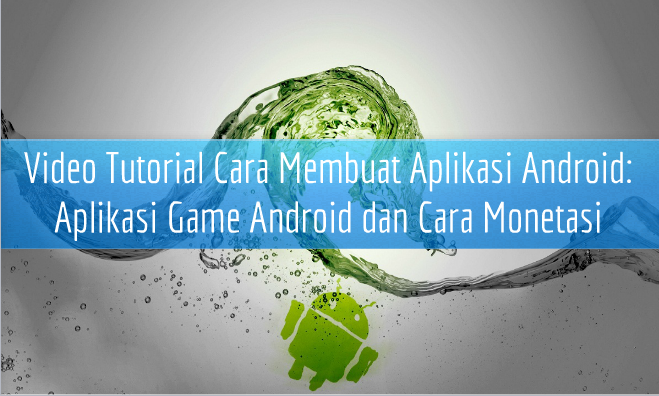

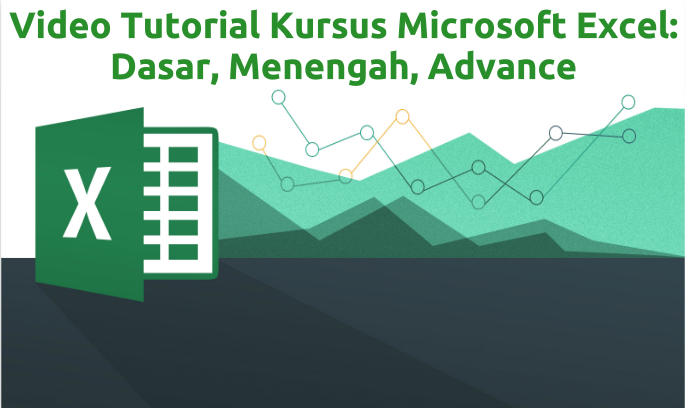

Tidak ada komentar:
Posting Komentar